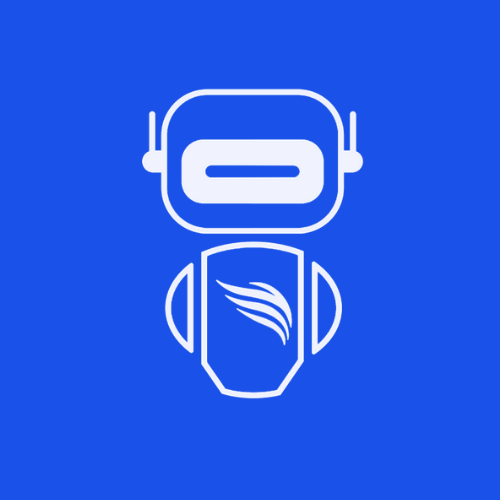
Innovative Machine Learning Model for Data Leak Detection
CybelAngel is able to alert on a set of sensitive blueprints amidst the thousands of billions of documents available on the web. Ever wondered how we are able to do it? Let us introduce you to the latest Machine Learning model for data leak detection: Content Scoring. We couple cutting-edge Machine Learning and human expertise. While nothing will replace cyber-analysts, technology can help lower the noise, speed up the detection of real threats, and contextualize the leak to facilitate the investigation. As CybelAngel deploys new detection capacities across file servers, we have drastically improved our Machine Learning algorithms used for filtering and contextualizing. Bottom-line, our customers can respond faster to the most critical leaks threatening their business.
How CybelAngel Uses Machine Learning For Data Leak Detection
Before appearing on a customer’s CybelAngel DRPS platform in the form of an Incident Report, a document detected on an open server has traveled across a funnel: At CybelAngel, Machine Learning is used for two main objectives. The first goal is to automatically detect the potential severity of an open server leaking documents, based on metadata like the file paths. This allows CybelAngel to scan the whole web quickly and efficiently. Our Machine Learning algorithms, trained on real-world, massive datasets, determine the likelihood that the server is critical. If this likelihood is above a certain threshold, the server is transmitted to cybersecurity experts for analysis. The second goal of Machine Learning is to detect relevant content inside the documents. It analyses the semantic context around the matching keywords to ensure the information is genuinely about our customers. The whole point of Machine Learning is turning thousands of matches into dozens of alerts before they can be forwarded to cyber-analysts for further investigation. In order to do so, we build, train, and use Machine Learning models such as Content Scoring.
About the Content Scoring Machine Learning model
Content Scoring is a proprietary Machine Learning model that looks into the content of detected documents on open file servers to decide whether they are a real threat. If so, it attributes a score to the alert based on criteria explained below. Content Scoring works in combination with other models in the Machine Learning pipeline, adding an extra layer of filtering and contextualization. Implementing Content Scoring has allowed us to:
- Reduce noise by 30% for Analysts;
- Push critical alerts for investigation before others;
- Ensure zero false positives;
- Avoid false negatives.
Read also: CISOs: Make 2020 the year you focus on third-party cyber risk
How does the Content Scoring Machine Learning model work?
The Content Scoring Machine Learning model takes input from two other algorithms that operate directly on the file text context to determine both file category and file sensitivity. Like other Machine Learning model, it consists of:
- A classifier, which aims at reading the content of the alert like a human would do. It predicts the criticality of a detection based on what it finds in the files. The classifier scores the detection from 1 to 100.
- A threshold, which is used to make decisions based on the score generated by the classifier.
Under a certain threshold, the alert will be discarded. It will never reach the analyst feed for investigation. Here is an example of what happens in the Machine Learning pipeline on an average day, for an average customer:
- 400 alerts for exposed document on open servers enter the Machine Learning pipeline;
- 100 out of those 400 will be analysed by the Content Scoring model, after 75% of the initial feed are discarded because the files’ metadata are not relevant.
- Only 70 alerts will eventually be sent to analysts for investigation.
This whole process happens in a matter of minutes. In a world where every second counts, our robust Machine Learning model gives customers a head start in securing unknowingly exposed assets.